Discover our scoring service.
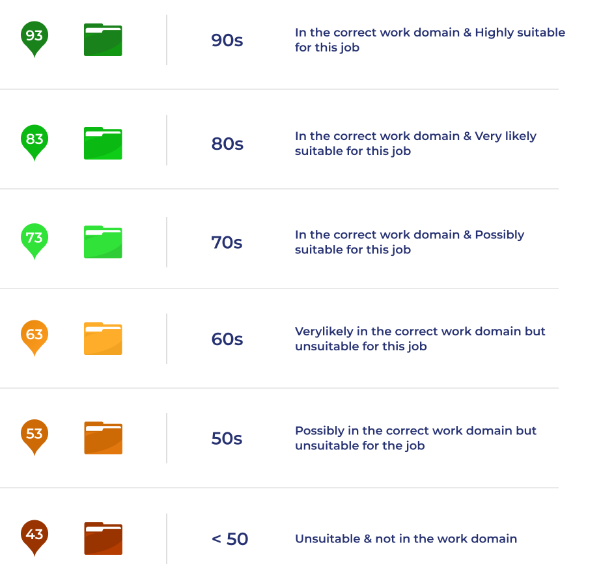
Geescore™ displays an AI based, validated, Jobseeker score, and provides a pdf download proof of the score, for submission to hiring managers.
Latest Developments - our 4th Gen Deep Transformer AI model.
Version 1.0
-
Latent Dirichlet Allocation (LDA) based topic model for scoring
-
Single RESTful API
-
Inputs –
o Job description extracted text
o Resume extracted Text
o Section text extracted from resume for skills, work experience, education, certification and awards
o Location – Zipcode / Postal code
-
Output:
-
Overall score,
-
section scores of
-
skills
-
work experience,
-
education,
-
certification,
-
awards / interest,
-
resume drop,
-
steady job,
-
total work experience,
-
location score (score based on distance from job location)
-
resume raw scoring – Entire JD vs Entire resume text
-
-
Version 2.0
-
LDA Scoring Model – Normalization updates for final score
-
Implemented Individual API split for all scoring component mentioned in the output of v1.0
-
Enhancements for improving response time
-
Moved Data processing to Parser
Version 2.1:
-
Fixes and Handler for scoring a Bad job (Job description does not have more than 40 words)
-
Fixes for LDA score > 100
-
Fixes for removing duplicate parsed data for each section of resume
Version 3.0:
-
Implemented RoBERTa based Deep Learning Transformer model to give similarity score for resume components – Skill score, Work experience score, Education score, Awards / Interests score, Certifications score, raw score
Version 3.1:
-
Implemented LDA Score for Skill score, Certification score, education score
-
Implemented RoBerta based transformer model similarity score for Work experience and Interests / Awards score
Version 4.0:
-
Updated to RoBERTa model for all components (skill, work experience, education, awards/certifications, interests)
-
Added weights to final scores of skill and work experience section
- Exploring further optimizations to improve performance speed
Scoring
We are pleased to share the science and business logic of the Geescore™Jobseeker Scoring solution. It is a dynamic hybrid approach to scoring based on constant improvement; lowering bias, increasing objectivity, and score validation. Currently we use a hybrid:
- Deep Transformer model for work experience, skills, education, working domain and
- Classic scoring algorithms, with 3 levels of matching science, as well as real-world recruiting parameters, for derived attributes such as job matches, steady job, location, etc.
How we reduce BIAS
We do not factor name, age, gender, country, country of work experience, country of education.